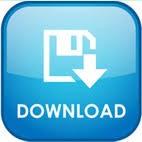
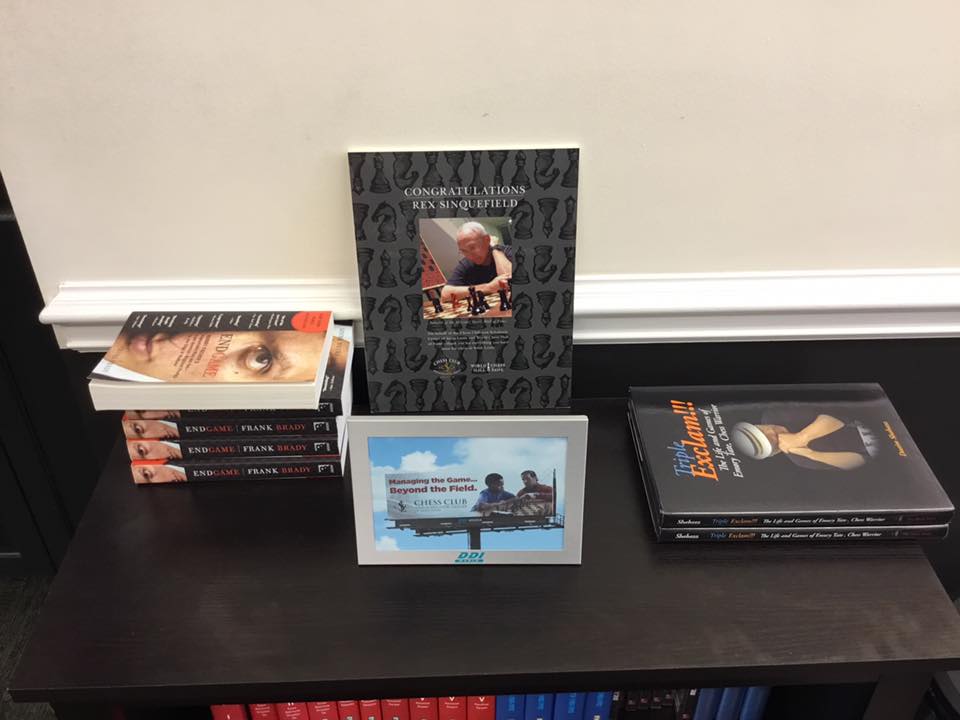
Solon Barocas, Anhong Guo, Ece Kamar, Jacquelyn Krones, Meredith Ringel Morris, Jennifer Wortman Vaughan, Duncan Wadsworth, and Hanna Wallach.Studying Up: Reorienting the study of algorithmic fairness around issues of power. Chelsea Barabas, JB Rubinovitz, Colin Doyle, and Karthik Dinakar.To Build a Better Future, Designers Need to Start Saying ‘No’. Beyond Bias: Re-Imagining the Terms of ‘Ethical AI’ in Criminal Law. CDC under scrutiny after struggling to report Covid race, ethnicity data. Fairness On The Ground: Applying Algorithmic Fairness Approaches To Production Systems. Chloé Bakalar, Renata Barreto, Miranda Bogen, Sam Corbett-Davies, Melissa Hall, Isabel Kloumann, Michelle Lam, Joaquin Quiñonero Candela, Manish Raghavan, Joshua Simons, Jonathan Tannen, Edmund Tong, Kate Vredenburgh, and Jiejing Zhao.”What We Can’t Measure, We Can’t Understand”: Challenges to Demographic Data Procurement in the Pursuit of Fairness. McKane Andrus, Elena Spitzer, Jeffrey Brown, and Alice Xiang.How Meta is working to assess fairness in relation to race in the U.S. Rachad Alao, Miranda Bogen, Jingang Miao, Ilya Mironov, and Jonathan Tannen.Global Partnership on Artificial Intelligence. Enabling data sharing for social benefit through data trusts. Aapti Institute and Open Data Institute.Towards this end, we assess privacy-focused methods of data collection and use and participatory data governance structures as proposals for more responsibly collecting demographic data. We argue that, by confronting these questions before and during the collection of demographic data, algorithmic fairness methods are more likely to actually mitigate harmful treatment disparities without reinforcing systems of oppression.
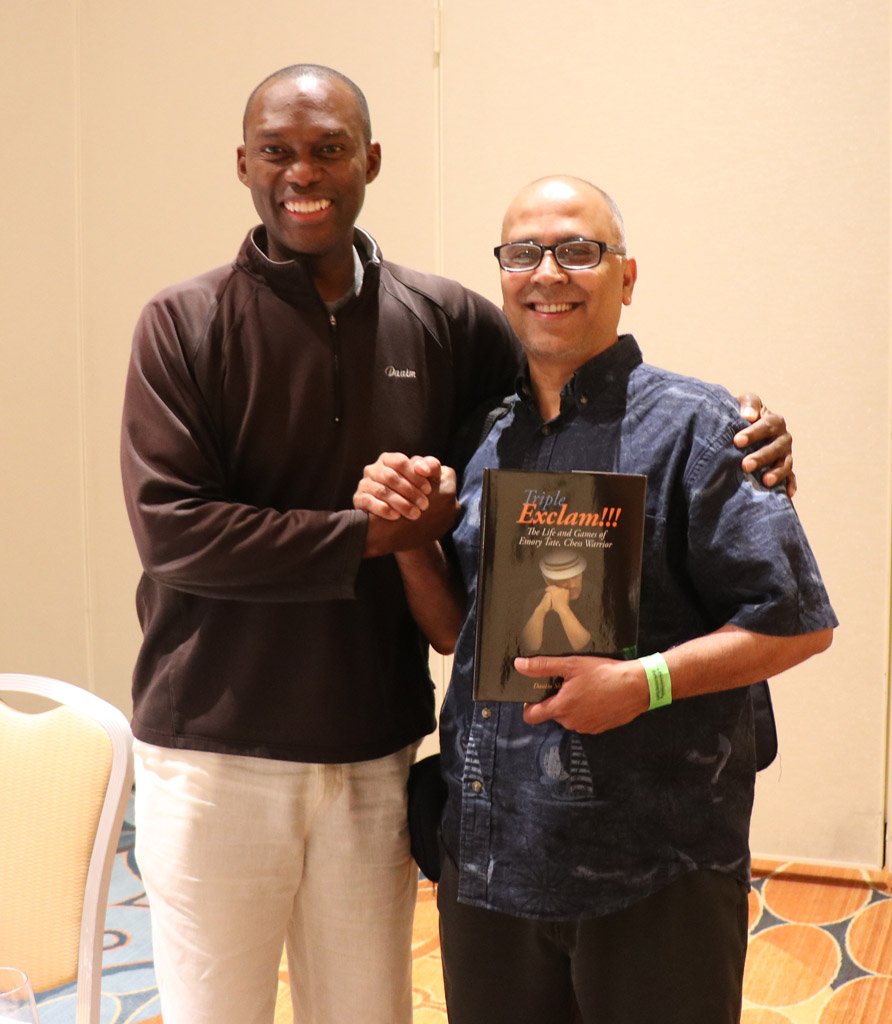
Looking more broadly, the risks to entire groups and communities include the expansion of surveillance infrastructure in the name of fairness, misrepresenting and mischaracterizing what it means to be part of a demographic group, and ceding the ability to define what constitutes biased or unfair treatment. For the risks to individuals we consider the unique privacy risks of sensitive attributes, the possible harms of miscategorization and misrepresentation, and the use of sensitive data beyond data subjects’ expectations. In this work, we explore under what conditions demographic data should be collected and used to enable algorithmic fairness methods by characterizing a range of social risks to individuals and communities. We show how these techniques largely ignore broader questions of data governance and systemic oppression when categorizing individuals for the purpose of fairer algorithmic processing.
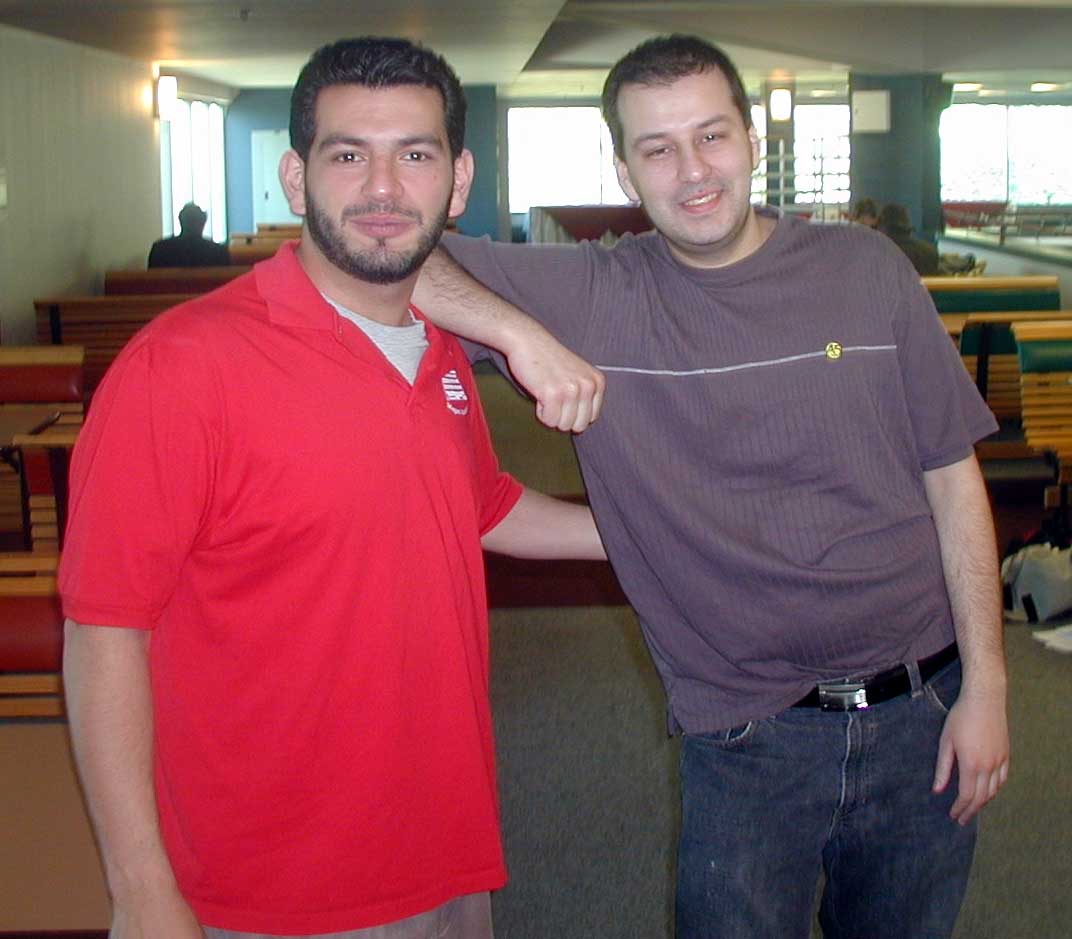
Through this paper, we consider calls to collect more data on demographics to enable algorithmic fairness and challenge the notion that discrimination can be overcome with smart enough technical methods and sufficient data. Most proposed algorithmic fairness techniques require access to demographic data in order to make performance comparisons and standardizations across groups, however this data is largely unavailable in practice, hindering the widespread adoption of algorithmic fairness.
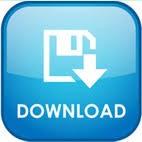